Duckietown
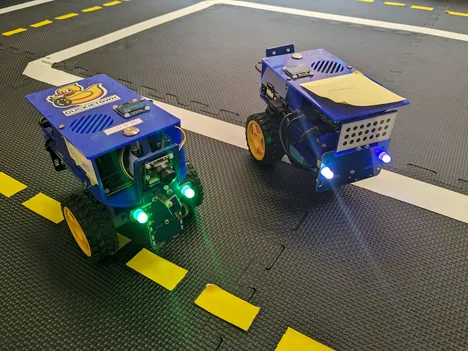
Duckietown, is an environment for studying autonomous driving in a scaled-down manner. It consists of Duckiebots that can move autonomously in a modular environment. The Duckiebots are robots consisting of sensors and motors controlled by an NVIDIA Jetson Nano.
We use this environment to visualize our research in the field of application specific MPSoCs. As a first use case we want to investigate and demonstrate the capabilities and the behavior of our hardware-optimized learning classifier tables (LCTs). These are rule-based reinforcement learning (RL) engines developed in our IPF project.
Initially, we analyze the image processing pipeline of the Duckiebots and apply our LCTs as additional controllers in the autonomous driving application of the Duckiebots. Based on the status of the Duckiebots, the LCTs should help to learn specific behaviors by influencing relevant parameters of the processing pipeline. In a first step, student works use a software version of the LCTs. In subsequent steps the Duckiebots should be extended by an FPGA board, which enables a hardware-based and independent realization of the LCTs.
Involved Researchers
How to get involved?
As we use Duckietown as a basis for our research and also to demonstrate results in the currently hot application domain of autonomous driving, there are plenty of opportunities for students to contribute.
You can get involved depending on your level of experience / study progress:
- Already during the bachelor phase you can practically apply your knowledge from LinAlg, StoSi and Regelungstechnik while you improve your coding skills and aquire knowledge about hardware architectures and autonomous driving. Get in touch with us, to talk about open tasks and to get access to our physical setup.
- Towards the end of your bachelor, it's the first time that you have to write a thesis. For that, have a look at open BA topics below.
The same applies for research internships (FPs) and master theses (MAs). - Depending on the currently planed steps, there might be tasks, which can't be assigned as BA, FP or MA. In such cases we also offer paied working student jobs. Open positions of that type are also listed below.
Thesis Offers
Interested in an internship or a thesis? Please send us an email.
The given type of work is just a guideline and could be changed if needed.
From time to time, there might be some work, that is not announced yet. Feel free to ask!
Assigned Theses
Duckietown - Improved RL-based Vehicle Steering
Beschreibung
At LIS, we try to leverage the Duckietown hardware and software ecosystem to experiment with our reinforcement learning (RL) agents, known as learning classifier tables (LCTs), as part of the Duckiebots' control system (https://www.ce.cit.tum.de/lis/forschung/aktuelle-projekte/duckietown-lab/).
More information on Duckietown can be found at https://www.duckietown.org/.
In previous work, an LCT agent to steer Duckiebots has been developed using only the angular heading error for the system state. In this Bachelor's thesis, the vehicle steering agent should be improved and its functionality extended.
Starting with the existing Python/ROS implementation of the RL agent and our image processing pipeline, multiple system parts should be enhanced. On the environment side, detecting the lateral offset from the center of a lane should be improved for reliability. This will require an analysis of the current problems and some adaptations in the pipeline, possibly some hardware changes.
With more reliable lane offset values, the agent's state observation can also include it, allowing us to move further from the default PID control towards a purely RL-based steering approach. This will involve modifications to the rule population, the reward function, and potentially the learning method. Different configurations are to be implemented and evaluated in terms of their resulting performance and efficiency.
The thesis aims to shift the vehicle steering entirely to the RL agent, ideally reducing the effort for manual parameter tuning while being comparable in driving performance and computation effort.
Voraussetzungen
- Experience with Python and, ideally, ROS
- Basic knowledge of reinforcement learning
- Structured way of working and problem-solving skills
Kontakt
michael.meidinger@tum.de
Betreuer:
Duckietown Bring-Up
Beschreibung
At LIS we want to use the Duckietown hardware and software ecosystem for experimenting with our reinforcement learning based learning classifier tables (LCT) as part of the control system of the Duckiebots: https://www.ce.cit.tum.de/lis/forschung/aktuelle-projekte/duckietown-lab/
More information on Duckietown can be found on https://www.duckietown.org/.
Towards this goal, we need a (followup) working student who is improving the current infrastructure.
Towards this goal, the following three major tasks are necessary:
- Developping an infrastructure to track and visualize measurement data of the platform (e.g. CPU utilization) as well as the executed application.
- During this task also the source and periodicity of already provided data should be analyzed.
- Setting up all Duckiebots incl. all their features and a pipeline to reflash them in case it's needed.
- FPGA-Extension: Searching for a concept, as well as implementing it.
- Final goal: demonstration of data exchange between NVIDIA Jetson and FPGA including protocol to specify the type of transfered data
Kontakt
flo.maurer@tum.de
Betreuer:
Completed Theses
Kontakt
michael.meidinger@tum.de
Betreuer:
Betreuer:
Kontakt
michael.meidinger@tum.de
Betreuer:
Kontakt
flo.maurer@tum.de
Betreuer:
Kontakt
flo.maurer@tum.de
michael.meidinger@tum.de
Betreuer:
Kontakt
flo.maurer@tum.de
michael.meidinger@tum.de
Betreuer:
Kontakt
flo.maurer@tum.de
michael.meidinger@tum.de
Betreuer:
Kontakt
flo.maurer@tum.de
Betreuer:
Kontakt
flo.maurer@tum.de
Betreuer:
Kontakt
flo.maurer@tum.de
Betreuer:
Kontakt
flo.maurer@tum.de
Betreuer:
Kontakt
flo.maurer@tum.de