Time-Sensitive Networking
Duration: | 3 years, 01.03.2021 – 30.05.2024 |
Partners: |
|
Project Lead: | Prof. Dr. Sebastian Steinhorst (sebastian.steinhorst(at)tum.de) |
Contact: | Rubi Debnath, MSc (rubi.debnath@tum.de) |
Project Objectives
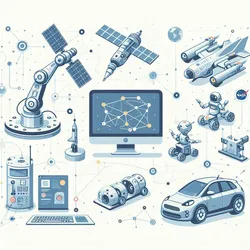
Time-Sensitive Networking (TSN) is a layer-2 technology standardized by IEEE 802.1. Time-Sensitive and Deterministic Networking is receiving significant interest from academia and industry. TSN aims to provide the necessary mechanisms for delivering time-critical and latency-sensitive data in industrial automation, automotive, and other industries where precise timing and low latency are crucial. TSN incorporates various IEEE standards, such as IEEE 802.1Qbv (time-aware shaper), 802.1Qbu/802.3br (frame preemption), 802.1Qca (per-stream filtering and policing), 802.1Qcc (stream reservation protocol), etc. These standards work together to ensure time synchronization, traffic scheduling, and network management capabilities.
The objective of this project is to develop an intelligent configuration framework for Time-Sensitive Networking (TSN) that enables dynamic adaptation and optimization of TSN network parameters and configurations in real-time. The goal is to enhance the flexibility, scalability, and performance of TSN systems by allowing them to adapt to changing network conditions, application requirements, and resource constraints during runtime. The project aims to design and implement algorithms for the configuration of large-scale industrial networks. The developed framework will be validated through experiments with extensive simulations to demonstrate its effectiveness in achieving dynamic adaptability and optimization in large-scale industrial networks.
Research Directions
- Optimized Scheduling Algorithms: Research is directed towards optimizing TSN scheduling and shaping algorithms to support mixed critical traffic types in various applications such as industrial automation, vehicular networks, aviation, and spacecraft. The developed algorithms are particularly tested on the Orion Test Case (NASA's Orion spacecraft) and industrial topologies.
- Scalability and Network Management: Ongoing research focuses on developing algorithms to deploy solutions for larger network topologies to tackle scalability issues. Deep reinforcement learning algorithms are successfully applied to develop solutions for large-scale networks.
- Interoperability and Standardization: For TSN to be widely adopted, it must seamlessly integrate with existing network protocols and devices. Research focuses on enhancing the interoperability of TSN with other networking standards and ensuring compliance with industry standards. The research includes not only standardized mechanisms but also new mechanisms that are ongoing for standardization.
- Machine Learning and AI Integration: The integration of machine learning and artificial intelligence into TSN is an emerging research direction. Research is ongoing to explore these technologies to predict network conditions, optimize resource allocation, and enhance fault tolerance.
Tools and Current Mechanisms of TSN
- TSN Simulator (TSNSimulate): Our simulation environment is built on top of OMNeT++ and INET 4.5.2. Since INET does not support all complex architectures and shaping mechanisms of TSN, we have implemented additional mechanisms to provide a comprehensive tool where all the different mechanisms of TSN can be evaluated in one place. Our tool supports a variety of mechanisms, including TAS, CBS, FP, ATS, CQF, CSQF, MCQF, and their combinations such as TAS+CBS, TAS+CBS+FP, TAS+ATS, TAS+ATS+FP, CQF+CBS, CQF+CBS+FP, CSQF+CBS, CSQF+CBS+FP, MCQF+CBS, and MCQF+CBS+FP.
- 5GTQ: Our open-source 5G-TSN tool called 5GTQ is the first to support full-fledged 5G-TSN simulation in a comprehensive TSN and 5G environment. Currently, our tool supports the TAS and CBS mechanisms in TSN. We have integrated a quality-of-service-aware mapping mechanism for the 5G-TSN network. 5GTQ is currently undergoing integration with official INET and Simu5G. Additionally, we are continuously working to add new features and enhancements to our framework.
Demo
Related Publications
2025
- [Preprint] Learning-Based Traffic Classification for Mixed-Critical Flows in Time-Sensitive Networking
Rubi Debnath, Luxi Zhao, Sebastian Steinhorst
IEEE International Conference on Communications (ICC 2025), Montreal, Canada - [Preprint] CyclicSim: Comprehensive Evaluation of Cyclic Shapers in Time-Sensitive Networking
Rubi Debnath, Luxi Zhao, Mohammadreza Barzegaran, Sebastian Steinhorst
IEEE Consumer Communications & Networking Conference (CCNC 2025), Las Vegas, NV, USA, 2025, pp. 1-9.
2024
- Quantifying the Impact of Frame Preemption on Combined TSN Shapers
Rubi Debnath, Philipp Hortig, Luxi Zhao, Sebastian Steinhorst
IEEE/IFIP Network Operations and Management Symposium (NOMS 2024), Seoul, South Korea, 2024, pp. 1-9.
2023
- 5GTQ: QoS-Aware 5G-TSN Simulation Framework
Rubi Debnath, Mustafa Selman Akinci, Devika Ajith, and Sebastian Steinhorst
2023 IEEE 98th Vehicular Technology Conference: VTC2023-Fall, Hong Kong, 2023, pp. 1-7. - Advanced Modeling and Analysis of Individual and Combined TSN Shapers in OMNeT++
Rubi Debnath, Philipp Hortig, Luxi Zhao, Sebastian Steinhorst
2023 IEEE 29th International Conference on Embedded and Real-Time Computing Systems and Applications (RTCSA), Niigata, Japan, 2023, pp. 1-10.
2022
- Quantitative Performance Comparison of Various Traffic Shapers in Time-Sensitive Networking
Luxi Zhao, Paul Pop, Sebastian Steinhorst.
In: IEEE Trans. Netw. Serv. Manag., 19(3), pp. 2899–2928. DOI: 10.1109/TNSM.2022.3180160
Contact Information
If you are interested in this topic, and you are looking for a bachelor's thesis, master's thesis, or research internship, feel free to contact: