- HW-Flow: A Multi-Abstraction Level HW-CNN Codesign Pruning Methodology. Leibniz Transactions on Embedded Systems (LITES), 2022 more… BibTeX
- AnaCoNGA: Analytical HW-CNN Co-design using Nested Genetic Algorithms. Design, Automation & Test in Europe Conference & Exhibition (DATE), 2022 more… BibTeX
- Accelerating and Pruning CNNs for Semantic Segmentation on FPGA. Design Automation Conference (DAC), 2022 more… BibTeX
- HW-FlowQ: A Multi-Abstraction Level HW-CNN Co-design Quantization Methodology. ACM Transactions on Embedded Computing Systems (TECS), 2021 more… BibTeX
- BinaryCoP: Binary Neural Network-based COVID-19 Face-Mask Wear and Positioning Predictor on Edge Devices. IEEE International Parallel & Distributed Processing Symposium, Reconfigurable Architectures Workshop (IPDPS-RAW), 2021 more… BibTeX
- Adversarial Robust Model Compression using In-Train Pruning. IEEE/CVF Conference on Computer Vision and Pattern Recognition Workshops (CVPRW), 2021 more… BibTeX
- Pruning CNNs for LiDAR-based Perception in Resource Constrained Environments. IEEE Intelligent Vehicles Symposium Workshops (IV Workshops), 2021 more… BibTeX
- Investigating Binary Neural Networks for Traffic Sign Detection and Recognition. IEEE Intelligent Vehicles Symposium (IV), 2021 more… BibTeX
- Hardware-Aware Mixed-Precision Neural Networks using In-Train Quantization. British Machine Vision Conference (BMVC), 2021 more… BibTeX
- Binary-LoRAX: Low-power and Runtime Adaptable XNOR Classifier for Semi-Autonomous Grasping with Prosthetic Hands. International Conference on Robotics and Automation (ICRA), 2021 more… BibTeX
- BreakingBED - Breaking Binary and Efficient Deep Neural Networks by Adversarial Attacks. Intelligent Systems Conference (IntelliSys), 2021 more… BibTeX
- OrthrusPE: Runtime Reconfigurable Processing Elements for Binary Neural Networks. 2020 Design, Automation & Test in Europe Conference & Exhibition (DATE), 2020 more… BibTeX
- L2PF - Learning to Prune Faster. International Conference on Computer Vision & Image Processing (CVIP), 2020 more… BibTeX
- Binary DAD-Net: Binarized Driveable Area Detection Network for Autonomous Driving. International Conference on Robotics and Automation (ICRA 2020), 2020 more… BibTeX
- ALF: Autoencoder-based Low-rank Filter-sharing for Efficient Convolutional Neural Networks. The Design Automation Conference (DAC), 2020 more… BibTeX
- An Efficient FPGA Accelerator Design for Optimized CNNs using OpenCL. ARCS Konferenz, 2019 more… BibTeX
- Resource-Aware Multicriterial Optimization of DNNs for Low-Cost Embedded Applications. Conference on Computer and Robot Vision, 2019 more… BibTeX
- DSC: Dense-Sparse Convolution for Vectorized Inference of Convolutional Neural Networks. Safe Artificial Intelligence for Automated Driving (SAIAD), 2019 more… BibTeX
MLOPs - Development, Optimization and Deployment of Machine Learning Algorithms on Embedded Hardware
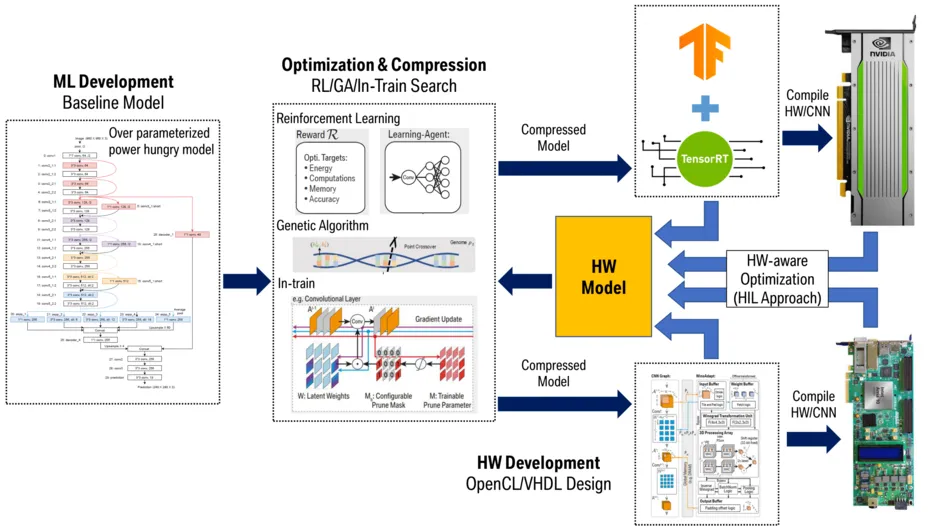
In recent years, convolutional neural networks (CNNs) have become the de facto standard for a variety of computer vision algorithms. However, their application in embedded systems such as autonomous driving or robotics raises major challenges. In particular, the targeted optimization of algorithms in terms of hardware efficiency, energy consumption, up to cost-optimized accelerators for edge inference of deep neural networks is an exciting research area.
Our research group is interested in HW/CNN co-design, a discipline which brings algorithm designers, toolchain and hardware architects together in the optimization of learning-based applications. Whether following a top-down, bottom-up, or meet-in-the-middle design paradigm, the hardware architecture must support parallel processing of the large number of convolutional operations, smart dataflows and reuse schemes, while lean and compressed neural network architectures must be optimized to enable the use of provisioned HW resources.
We are fully committed to researching methods and techniques aimed at reliably and efficiency in deploying machine learning models on embedded systems. MLOPs are our focus here for data-driven optimization and application of neural networks in embedded systems. If you want to be part of the transformation, take a look at the seminar and thesis topics below.